Add context from private data and documents to GenAI LLMs to reduce hallucinations and increase performance through Retrieval Augmented Generation.

Key use cases for Large Language Models include:
- Generation: LLMs can be used to generate text, translate languages, write different kinds of creative content, and answer your questions in an informative way. For example, LLMs can be used to generate realistic dialogue for chatbots, write news articles, or even create poems.
- Summarization: LLMs can be used to summarize text, extract the main points of an article or document, and create a shorter version that is still accurate and informative. For example, LLMs can be used to summarize research papers, news articles, or even books.
- Classification: LLMs can be used to classify text, identify the topic of a document, and determine whether it is positive or negative, factual or opinion, etc. For example, LLMs can be used to classify customer reviews, social media posts, or even medical records.
- Extraction: LLMs can be used to extract information from text, identify specific entities or keywords, and create a table or list of the extracted information. For example, LLMs can be used to extract contact information from a business card, product information from a website, or even scientific data from a research paper.
- Q&A: LLMs can be used to answer questions in an informative way, even if they are open ended, challenging, or strange. For example, LLMs can be used to answer questions about a particular topic, provide customer support, or even generate creative text formats of text content.
While Generative AI Large Language Models often seem like a panacea, they suffer from a number of key issues:
- Hallucinations: models will ‘make stuff up’ if they don’t know an answer. They also suffer from a lack of contextual understanding. Techniques like few-shot prompting can help.
- Inference Performance: even the faster models are slower than a dial-up modem, or a fast typist! They also suffer from latency or time to first token. For most queries, expect 10–20 second response times from most models, and even with streaming, you’ll end up waiting a few seconds for the first token to be generated!
- Inference Cost: LLMs are expensive to run! Some of the top 180B parameter models may need as many as 5xA100 GPUs to run, while even quantized versions of 70B LLAMA would take up a whole GPU! That’s one query at a time. The costs add up. For example, a dedicated A100 might cost as much as $20K a month with a cloud provider! A brute force approach is going to be expensive.
- Stale training data: even top models haven’t been trained on ‘recent’ data, and have a cut-off date. Remember, a model doesn’t ‘have access to the internet’. While certain ‘plugins’ do offer ‘internet search’, it’s just a form of RAG, where ‘top 10 internet search query results’ are fed into the prompt as context, for example.
- Use with private data: LLMs haven’t been trained on *your* private data, and as such, cannot answer questions based on our dataset, unless that data is inject through fine tuning or prompt engineering.
- Token limits / context window size: Models are limited by the TOKEN_LIMIT, and most models can process, at best, a few pages of total input/output. You can’t feed a model and entire document, and ask for a summary or extract facts from the document. You need to chunk documents into pages first, and perform multiple queries.
- They only support text: while this sounds obvious (from the name), it also means you can’t just feed a PDF file or WORD document to a LLM. You first need to convert that data to text, and chunk it to fit in the token limit, alongside your prompt and some room for output. Conversions aren’t perfect. What happens to your images, or tables, or metadata? It also means models can only output text. Formatting the text to output HTML or DOCX or other rich text formats requires a lot of heavy lifting in our pipeline.
- Lack of transparency / explainability: why did the model generate a particular answer? Techniques such as RAG can help, as you are able to point at the ‘context’ that generated a particular answer, and even display the context. While the LLM answer may not necessarily be correct, you can display the source content that helped generate that answer.
- Potential bias, hate, abuse, harm, ethical concerns, etc: sometimes, answers generated by an LLM can be outright harmful. Using the RAG pattern, in addition to HARM filters can help mitigate some of these issues.
- Training and fine tuning costs: to put it in perspective, a 70B model like LLAMA2 might need ~2048 A100 GPUs for a month to train, adding up to $20–40M training cost, not to mention what it takes to download and store the data. The: “Training Hardware & Carbon Footprint” section from the LLAMA2 paper suggests a total of 3311616 GPU hours was used to train LLAMA2 (7/13/34 and 70B)!
It helps to think of of Large Language Models (LLMs) like mathematical functions, or your phone’s autocomplete:
f(x) = x’
- Where the input (x) and the output (x’) are strings. The model starts by looking at the input, then will ‘autocomplete’ the output.
- For example, f(“What is Kubernetes”) = “Kubernetes, often abbreviated as K8s, is an open-source platform designed to automate deploying, scaling, and operating application containers.”
- Most chat interfaces will also provide a default system prompt. For LLAMA2, this is: “
You are a helpful, respectful and honest assistant. Always answer as helpfully as possible, while being safe. Your answers should not include any harmful, unethical, racist, sexist, toxic, dangerous, or illegal content. Please ensure that your responses are socially unbiased and positive in nature. If a question does not make any sense, or is not factually coherent, explain why instead of answering something not correct. If you don't know the answer to a question, please don't share false information."
- Depending on the model and interface, there may be ‘hidden’ inputs to your model. Many Chat interfaces will include a conversational memory, where they insert a moving window of your previous prompts into the current prompt, as context. It would look something like this: “Below are a series of dialogues between a user and a AI assistant…. [dialogues] [new content]“
The inputs to a model are a little more complex though:
f(training_data, model_parameters, input_string) = output_string
- training_data represents the data it was trained (different models will provide different answers). While not an ‘input’ as such, the data the model was trained (and how it was trained) on plays a key factor in the output.
- model_parameters represent things like “temperature”, “repetition penalty”, “min tokens” or “max tokens”, “top_p”, “top_k”, and other such values.
- input_string is the combination of prompt and context you give to the model. Ex: “What is Kubernetes” or “Summarize the following document: ${DOCUMENT}”
- the ‘prompt’ is usually an optional instruction like “summarize”, “extract”, “translate”, “classify” etc. but more complex prompts are usually used. “Be a helpful assistant that responds to my question.. etc.”
- The function can process a maximum of TOKEN_LIMIT (total input and output), usually ~4096 tokens (~3000 words in English, fewer in say.. Japanese). Models with larger TOKEN_LIMITS exist, though they usually don’t perform as well above the 4096 token limit. This means, in practice, you can’t feed a whole whitepaper to an LLM and ask it to ‘summarize this document’, for example.
What Large Language Models DON’T DO
Learn: A model will not ‘learn’ from interactions (unless specifically trained/fine-tuned).
Remember: A model doesn’t remember previous prompts. In fact, it’s all done with prompt trickery: previous prompts are injected. The API does a LOT of of filtering and heavy lifting!
Reason: Think of LLMs like your phone’s autocomplete, it doesn’t reason, or do math.
Use your data: LLMs don’t provide responses based on YOUR data (databases or files), unless it’s include in the training dataset, or the prompt (ex: RAG).
Use the Internet: A LLM doesn’t have the capacity to ‘search the internet’, or make API calls.
- In fact, a model does not perform any activity other than converting one string of text into another string of text.
- Any 3rd party data not in the model will need to be injected into prompts (RAG)
Adding a LLM to your software architecture:
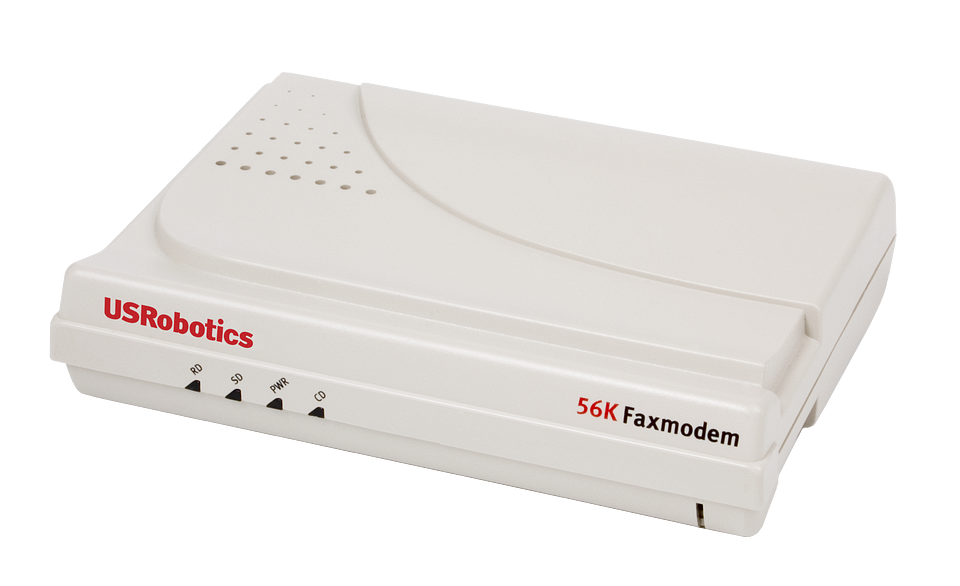
Believe it or not, LLMs are much slower than even a faxmodem! At WPM = ((BPS / 10) / 5) * 60, a 9600 baud modem will generate 11520 words / minute.
At an average 30 tokens / second (20 words) for LLAMA-70B, you’re getting 1200 words / minute!
Large models (70B) such as LLAMA2 can be painfully slow. Smaller models (20B, 13B, 7B) are faster, and require less GPU to run. Quantized models are also faster, but provide lower quality responses.
Quantize your model for faster inference
You can load and quantize your model in 8, 4, 3 or even 2 bits, sacrificing quality for faster inference speed.
This is always a tradeoff, as you’re sacfificing model output quality for faster inferencing. Since a quantized model needs less GPU VRAM to run in, this helps you run large models on commodity hardware.
Reducing model hallucinations:
LLMs lack context from private data — leading to hallucinations when asked domain or company-specific questions. RAG can help reduce hallucinations by ‘injecting’ context into prompts. Papers:
- Retrieval Augmented GFeneration as a mechanism to reduce hallucinations
- Retrieval Augmentation Reduces Hallucination in Conversation
- Detecting and Preventing Hallucinations in Large Vision Language Models
- Chain-of-Thought Prompting Elicits Reasoning in Large Language Models
- Tree of Thoughts: Deliberate Problem Solving with Large Language Models
- Halo: Estimation and Reduction of Hallucinations in Open-Source Weak Large Language Models
Retrieval Augmented Generation and the importance of Vector Databases
A vector database is a specialized database designed to store and query vector embeddings efficiently. Vector embeddings are numerical representations of text, images, audio, or other data. They are used in a variety of machine learning applications, such as natural language processing, image recognition, and recommendation systems.
Near Vector search or how to Search for “Sky” and find “Blue”:
- Finding the most similar documents to a given document
- Finding documents that contain a specific keyword or phrase
- Clustering documents together based on their similarity
- Ranking documents for a search query
Popular vector databases include ChormaDB, Weaviate, Milvus.
Advantages of using a VectorDB with your LLM, in a Retrieval Augmented Generation Pattern:
- Insert your data into prompts every time
- Cheap, and can work with vast amounts of data
- While LLMs are SLOW, Vector Databases are FAST!
- Can help overcome model limitations (such as token limits) — as you’re only feeding ‘top search results’ to the LLM, instead of whole documents.
- Reduce hallucinations by providing context.
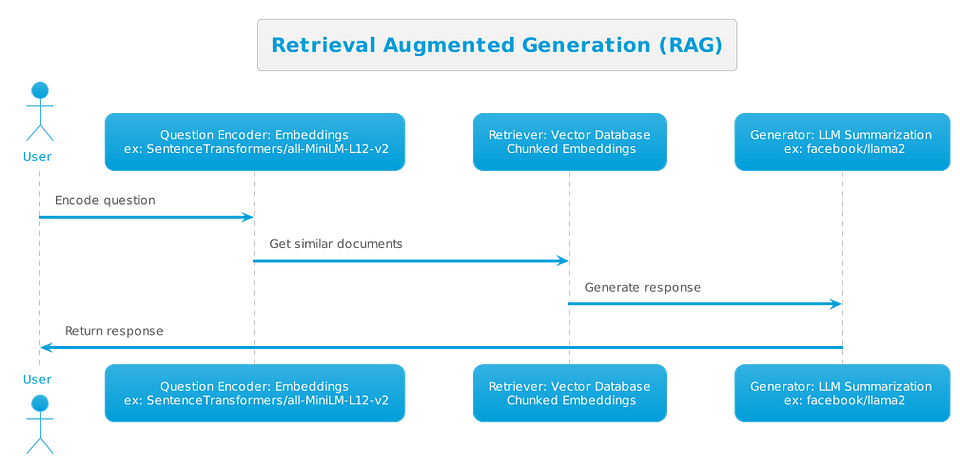
Loading Documents into your Vector Databases:
Loading data into your vector database typically requires you to convert documents to text, split the text into chunks, then vectorize those chunks using an embedding model. SentenceTransformers offers a number of pre-trained models, such as all-mpnet-base-v2 or all-MiniLM-L12-v2 that perform well for English text.
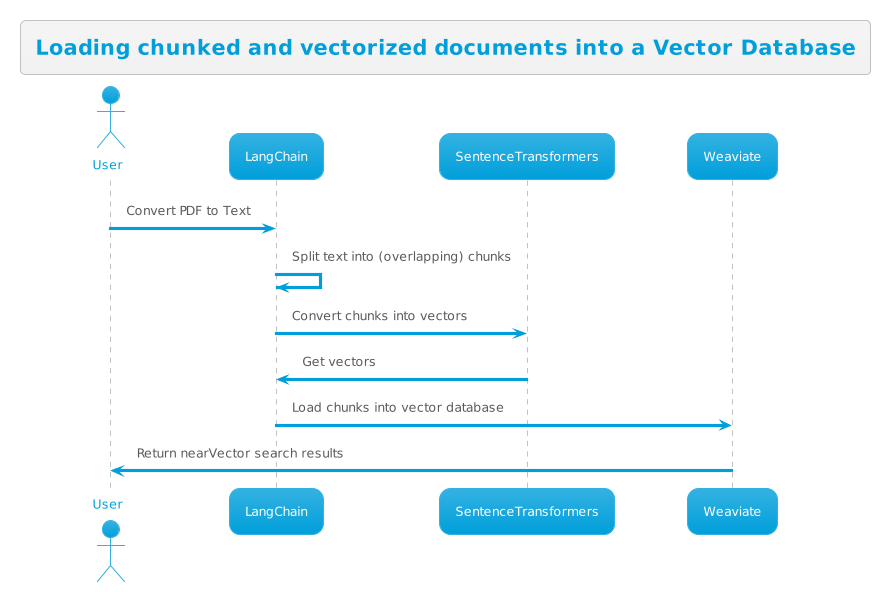
Scaling factor for RAG: what to consider:
- Vector Database: consider sharding and High Availability
- Fine Tuning: collecting data to be used for fine tuning
- Governance and Model Benchmarking: how are you testing your model performance over time, with different prompts, one-shot, and various parameters
- Chain of Reasoning and Agents
- Caching embeddings and responses
- Personalization and Conversational Memory Database
- Streaming Responses and optimizing performance. A fine tuned 13B model may perform better than a poor 70B one!
- Calling 3rd party functions or APIs for reasoning or other type of data (ex: LLMs are terrible at reasoning and prediction, consider calling other models)
- Fallback techniques: fallback to a different model, or default answers
- API scaling techniques, rate limiting, etc.
- Async, streaming and parallelization, multiprocessing, GPU acceleration (including embeddings), generating your API using OpenAPI, etc.
- Retraining your embedding model
RAG Talk from Shipitcon can be found on GitHub and YouTube:
- https://github.com/crivetimihai/shipitcon-scaling-retrieval-augmented-generation
- https://www.youtube.com/watch?v=lL4DPcxljH8
Social media
- https://twitter.com/CrivetiMihai — follow for more LLM content
- https://youtube.com/CrivetiMihai — more LLM videos to follow
- https://www.linkedin.com/in/crivetimihai/
0 comments:
Post a Comment